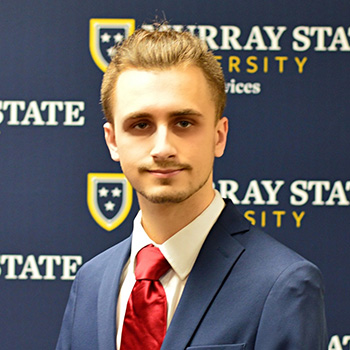
Austin Coursey, student
By Alex Pologruto | Mar 31, 2022
MURRAY, Ky. — A team of researchers at Murray State University, led by Dr. Saptarshi Sengupta, has advanced the state-of-the-art in prognosticating the remaining useful life (RUL) of Hard Disk Drives (HDD) by using deep Long Short Term Memory (LSTM) Networks which are a special type of recurrent neural networks well suited for temporal prediction tasks. The title of the team’s published paper is ‘Remaining Useful Life Estimation of Hard Disk Drives using Bidirectional LSTM Networks.’
Predicting impending failures in safety-critical applications is key to maintaining continued uptime and avoiding loss of revenue. In this context, the use of Artificial Intelligence (AI) and Deep Learning (DL) are emerging to be a clear go-to-choice for researchers practicing in the field.
The research team consisted of Austin Coursey, Dr. Gopal Nath, and Dr. Saptarshi Sengupta, all from Murray State University and Dr. Srikanth Prabhu, from Manipal Institute of Technology in India.
Coursey, a senior undergraduate student in the Department of Computer Science and Information Systems from Russellville, Kentucky, who is also the first author on the study, is a recipient of the Outstanding Senior of the year 2021 in the CSIS department. His research interests span Prognostics and Health Management using Machine Learning.
Nath is an assistant professor of Mathematics and Statistics who works in Machine Learning, Biostatistics and Bioinformatics.
Sengupta is an assistant professor of Computer Science and Information Systems and his research areas include large-scale Cyber Physical Systems, Prognostics, Deep Learning and Optimization.
Prabhu is an associate professor of Computer Science and Engineering at Manipal Institute of Technology in India who works with Big Data Analytics and Software Engineering.
Austin Coursey, student
Dr. Gopal Nath
Dr. Saptarshi Sengupta
Dr. Srikanth Prabhu
“My research uses data from physical devices, such as hard-disk drives or aircraft engines,” said Coursey. “We train a machine learning model on this data to learn to predict the remaining useful life of one of these devices. By having an accurate estimate of the remaining useful life, the reliability of these devices and larger systems that use these devices can be improved.”
The collaboration has led to an improvement in estimating the remaining useful life of hard disk drives by reducing the error of prediction for failure horizons spreading out as far as 60 days before failure. This implies that maintenance personnel may have more time to take mitigating steps to avoid system downtime. The group used a Bidirectional LSTM Network with sophisticated feature standardization mechanisms to achieve the superior results on the set of HDD data used for the work. The research has been published in IEEE Big Data 2021, a leading international conference on big data. The research paper can be accessed from IEEE Xplore at https://bit.ly/36DPcnB.